The Crimson Permanent Assurance Gets Upskilled
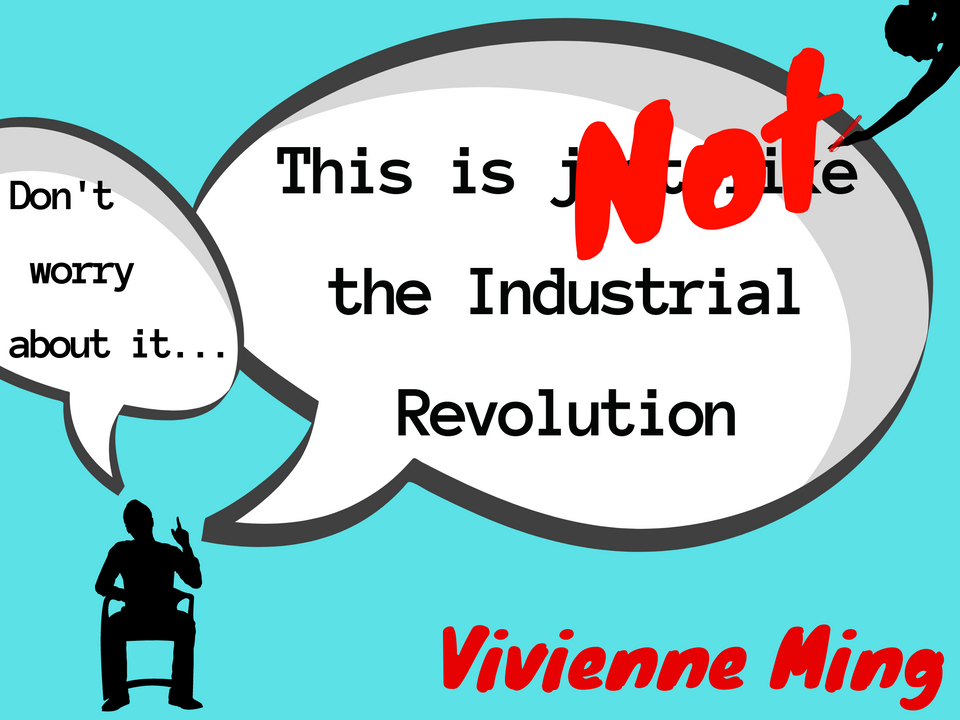
“You should read the World Economic Forum report on how AI will empower everyone.”
– Pompous Mansplainer
Creative complementarity produces overwhelming benefits to everyone involved, while substitution via automation barely moves the needle. So it’s an easy choice, right? If it were easy for individuals, then all of the teachers, recruiters, doctors, lawyers, and others we’ve analyzed so far, along with the 80,000 hypothetical employees from our original thought experiment, would have very different jobs than they do today. Pretending that individual choice is a magic wand77that can solve this problem divorces organizations from both their influence on their communities and their interest in cultivating creative talent to fill rising demand.
For most companies, this isn’t any easier a choice than it is for individuals. For the last several years, I’ve moderated a corporate war-gaming exercise on “courageous decision-making” in which high-level employees from Fortune 500 companies were confronted with choices that might be summarized as the “right” vs. the “righteous” (e.g. firing idle employees during a global pandemic vs. paying them to take classes from home). When these highly educated and powerful individuals made decisions in the abstract, most couldn’t even contemplate choosing the right over the righteous. Yet when they had stakes in the game, “right” dominated78.
As it turns out, we don’t have to rely on an abstract game to tell us how organizations are responding today; they’ve been making these choices for decades. There are significant costs and uncertainty in the “righteous” choice of completely retooling your company to take advantage of creative complementarity. The overwhelming trend over the last 40 years has been deprofessionalization.
Vivienne Needs a Loan
Take, for example, a loan application. In 2019, the US financial industry received 15.1 million home loan applications with 9.3 million resulting in loans. I’ve applied for a few loans in my lifetime. In fact, a couple years ago, one of my companies needed a solution to a truly absurd problem. The company’s earnings were cyclical and tended to be a bit low during the winter, and so we built a nest egg through the summer and fall in preparation to cover payroll. Unfortunately for us, taxes are calculated right in the middle of this period, which means that an organization designed to be zero profit nonetheless looked like it was carrying a big positive balance every year, a balance the IRS most definitely wanted its share of. The simplest solution was a line of credit. Despite the seemingly obvious virtue of saving instead using credit, the system forced us to spend down all of our capital at the end of the year and take out a recurring loan to cover salaries. The cost of dealing with the bank was simply much less than dealing with the IRS, which is why whole industries exist to avoid payments to governments, legitimate or otherwise79.
The application process was shockingly painless. I went online, answered some questions, and hit submit. Not too long ago, I would have walked into a bank in person and talked with some unfortunate soul in a cubicle whose eyes screamed, “rescue me”. They might have tried to upsell me on various banking products before sending my application up the food chain for initial approval. From there, it would have been faxed to an office where teams of analysts would process all of my financial data by hand. Depending on the era80, they would then either crank a calculator or boot up a computer and run a bunch of models to project how likely I am to pay back my loan. From that projection, these faceless analysts and their models would decide how much credit I should be offered and at what rate. My form would bounce around a few more cubicles, go to a higher level loan officer for approval, and then back to my local branch where my banker would deliver the final judgment. I would then get a call on my landline and go back into the bank to sign my form.
The modern version of this process is conceptually the same, except I didn’t have to go anywhere81 and no one had to punch numbers on my behalf. My credit information flew from one branch to another with a single click and likely showed up on the computer screen of someone in Mumbai or Bangalore who ran a standardized risk assessment analysis. I did get a call from someone doing a little upselling, but the bank had already decided to give us the line of credit.
In a globally connected world, paying to share a zipcode with someone I'll never meet doesn’t make any sense.
Multiple forces have transformed the process of assessing a loan application. Deprofessionalization is at play via sophisticated analytics models, but the two dominant factors over the last 40 years have been outsourcing and robotic process automation82. The latter, quite reasonably, does away with all of the absurdities of data entry and faxing. The former is the inevitable outcome of a very simple reality: the median university-trained analyst in India is just as talented as their competition in the US and the UK but less expensive. In a globally connected world, paying to share a zipcode with someone I'll never meet doesn’t make any sense.
And so, an outsourced analyst in Mumbai receives my data via robotic process automation and performs a deprofessionalized risk analysis using standardized risk assessment software. The net result of these “choices” is that I get fast, painless access to credit and several highly trained jobs drift from The Deprofessionalized to The Downskilled.
My experience with the rapid change in the financial industry isn’t restricted to being a customer. A dozen different banks have asked me to brief their boards and executives on AI and the future of work. Automation is having huge impacts all over the financial sector, from financial analysts to tax accountants to bank tellers. Despite the entire body of my work being a call to invest in human capacity, nearly every bank responded to my briefings with, “Wonderful, wonderful… Thank you so much, that was wonderful… By the way, can you build a chatbot to replace all of our tellers?” It turns out that the companies building AI products aren’t the only ones selling the AI bait-and-switch. Every major employer claims that no one will be laid off. They say that AI will make the company and its products better, but more importantly, it will make employees better. “Don’t worry about it! It’s just like the Industrial Revolution, but with health insurance.”
Their human capital is their advantage83, an advantage they lose if they trade it all away for a chatbot.
Few big companies are willing to publicly admit that automation will have a negative impact on their workforce, and in fact often claim the exact opposite. But behind the scenes, that’s all that they talk about. I visit a wide range of companies all over the world–Asia, Africa, Europe, the US, South America—and nearly every one asks me how they can use AI to substitute for what they see as a costly, low-impact human workforce. The banks, in particular, feel an existential threat from the technology industry. Alibaba, Amazon, Tencent, Microsoft, and all of the other big players are pushing into their markets with app-based financial products. The financial industry has spent 40 years chasing lower labor costs via outsourcing and offshoring; is it really a surprise that they mistake the tech industry’s lower labor costs as its biggest advantage?
Their human capital is their advantage83, an advantage they lose if they trade it all away for a chatbot. These banks want to fight a technology war against the ten biggest technology companies in the world, who also happen to be the very companies selling the chatbots the banks intend to use. It’s like saying, “I’m going to buy guns from China to invade China.”
There is an obvious dissonance between what these companies acknowledge in public and what they are actually considering in private: bait in public, switch in private. There are a handful of senior executives at every company that actually want to make a positive impact in their employees' lives, but there are relatively few organizations that have made this a policy priority. Some of them might surprise you: every military I’ve briefed wants to know how to make super soldiers (and yes, I’m sure for some Deathlok is what they are talking about) but they are also genuinely interested in what technology can do for the cognitive and emotional capacity of their workforce.
Crimson Flags
A company that has caught my attention is the one that was simultaneously the most brutally honest about the future of its workers and yet the most aspirational about what could be done. Rather than disclose my noble collaborators, we will simply refer to them as the Crimson Permanent Assurance Company. They employ nearly half a million people with diverse, technologically-sophisticated operations spanning the globe. They shared with me that they projected 200,000 layoffs over the next ten years due to “technological obsolescence”. This wasn’t about business downturn or unqualified employees; rather, every forecasting model told them that the automation of those jobs was inevitable, regardless of how well-educated and intelligent the employees were. And most of those 200,000 layoffs would be in India.
The globalization of professional services is based on efficiency as a solution...why not choose the cheaper option when it comes along?
The reason so many multinational companies are now potentially facing massive layoffs in countries like India is the same reason they have so many employees there in the first place: lower costs for cognitive labor. The value of outsourcing and offshoring comes from the fact that the same caliber of human talent simply costs less in India than it does in the US. The globalization of professional services is based on efficiency as a solution. Jobs in this sector of the economy have been structured this way very intentionally. The cognitive labor of a deprofessionalized credit analyst is almost entirely rote, and it doesn’t necessarily matter who does it. So why not choose the cheaper option when it comes along?
And there are so many options available. With the addition of cheap AI automation, businesses have four sources of labor to do the same deprofessionalized job: expensive employees in highly developed urban centers; less expensive offshored employees; highly flexible outsourced contractors; and, the (in theory) least expensive AI “labor pool”. In a basic sense it’s possible to think of automation as just another competitor in the job market. In manufacturing, for example, each individual factory line robot has the effect of suppressing wages and increasing unemployment just as if 3.5 additional human workers84 had entered the local job market. When substitution defines a labor market, cheaper dominates over better. In an increasing number of tasks, AI is undeniably cheaper than people.
What makes the Crimson Permanent Assurance Company different from so many others I’ve briefed isn’t just that they were honest about their projected layoffs, but that they believed those employees had an untapped potential worth keeping. What they hoped we could discover was the means of realizing that potential. To be clear, the people they projected to lay off weren’t dishwashers or coal miners; they weren’t even construction equipment operators. These were knowledge-economy, cognitive labor professionals. You’d think that keeping them employed would be easy–why not just turn around and reskill them?
My first interaction with the swashbuckling pirates of Crimson Permanent Assurance came at CES, the annual Vegas tribute to all the worst things about technology marketing…absolutely useless. Imagine a plastic version of Monaco filled with 500,000 Glengarry Glen Ross characters all selling 3D TVs and app-based home juicing machines85. But to my surprise, the particular panel to which I was invited was actually fun. The participants and I got to talk shop about artificial intelligence beforehand, and everyone had genuinely interesting things to say. We were all pumped up like nerdy rockstars, but as we walked out on stage, most of my funny, thoughtful, and engaging co-panelists were secretly substituted with marketing-approved, lookalike talking-point-bots86.
Still, it’s a blast being the one demented mad scientist not beholden to the man at an otherwise crushingly mainstream conference. Perhaps that’s what made me stand out to Crimson’s crew: their head of HR, Chief Strategic Officer, director of their innovation hub, and even their top swashbuckler. Each came to me independently talking in different ways about their labor challenges and how much they wanted a different choice than layoffs or costly churn.
While most of Crimson’s projected layoffs were in India, the choices around the future of work are global. China, Ukraine, Indonesia, and many other countries have transitioned large parts of their economies towards providing cheap cognitive labor to the rest of the world87. This was a huge economic step forward from the marginal value of the cheap physical labor that had been the focus of many underdeveloped economies. The devastating volatility of so many past industrial revolutions had demonstrated that a low-cost labor force wasn’t competitive to highly automated production lines. And so these countries began investing heavily in education and infrastructure to build a large and robust, if still largely routine, cognitive labor pool. They did everything they were “supposed to do” to prepare for the future of work. If only keeping these promises was as easy as making them.
AI-based automation is already slowing growth in economies that have invested in churning out “cheap” cognitive labor...
But this isn’t just another Industrial Revolution. The foundation of these new jobs is no longer low-skill physical labor, but that’s not what’s being automated. Just as physical automation devastated economies over much of the world in the 19th and 20th centuries, AI-based automation is already slowing growth in economies that have invested in churning out “cheap” cognitive labor and will eventually drive that growth negative. We already see that greater automation drives greater reshoring88 (and even in developed economies reshoring suppresses low-skill wages while favoring creative labor). The World Bank projects that “labor-saving technologies coupled with reshoring may disrupt labor markets in developing countries and result in job losses.” This was already happening pre-Covid-19 to the offshored and outsourced workforce in the Philippines, and the Brooking Institute warns of the same reversal of productivity and human capacity gains in Africa. If you’re still wondering about my loan application, PWC expects AI to “unleash a wave of reshoring as [finance] firms discover that this approach is even cheaper than outsourcing or offshoring.” If the tasks are routine, you won’t have labor cheaper than AI.
This isn’t just a problem in developing countries; in all economies, rote jobs across skill levels will undergo substitution of all kinds. We can’t keep the promise of university education as a path to a global professional middle-class, because it turned out that Crimson Permanent Assurance couldn’t even keep that promise to some of its most highly trained employees.
Within the Crimson, there was a 1000-person team dedicated to an arcane sector of the finance world. A couple of years before our collaboration began, a change in the market caused this line of work to disappear almost overnight. The Crimson Permanent Assurance Company could have looked at these 1000 people as a redundant waste of salary, but instead, they thought they’d won the lottery. They had 1000 elite, enculturated employees free to sail the most lucrative new markets in finance. So, instead of laying them off, they decided to upskill them all.
Crimson HR experts based their upskilling plan on a “neighborhood” model of skills. These models embed skill relationships and current patterns in networks which map out the similarity in skills across all jobs. The very reasonable assumption behind upskilling is that it should be easier to retrain a worker towards a very similar set of skills, i.e. those that are closest in the network to their current skill set. For example, in 2019 Amazonpledged to upskill 100,000 US employees into its “fastest growing highly-skilled jobs…data mapping specialists, data scientists, solutions architects, and business analysts.” From fulfillment centers to data science via some upskilling classes on Python and EC2—what a dream!
During my visits to Crimson Permanent Assurance, I met a tall, lanky German fresh out of academia developing an upskilling tool for the company. He started with a neighborhood model but then analyzed internal and external data to identify which skills were “dying” and which were predicted to take off in the next ten years. For example, he found that demand for analysts combining SAP and finance expertise was dropping, but demand pairing SAP with oil and gas industry experience was taking off. So, these neighboring skill sets had a big value gradient; retraining these financial analysts into energy analysts was potentially a huge gain. You can see how this parallels the farm to factory example–for the individual employee, it’s a lateral transition that involves significant work simply to maintain the same place within a company. They were providing the same kind of routine professional services and even using the same tools but in a different industry with greater value to the company.
Applying the hot skills tool to Crimson’s 1000-person team, with their esoteric financial expertise gained through elite university education and years of experience, revealed a variety of neighboring roles in the financial industry to which they could be upskilled89. This led to much spending of money and development of a boutique upskilling program. The Crimson couldn’t ask for a better group to upskill than a bunch of elite university grads moving from one sector of finance to another. It was a golden opportunity.
Two years later, the number of members from the original team still working for the company had dropped to single-digits. In short, 1000 highly-trained employees that the company actively wanted to preserve and promote had essentially given up. Having spent years becoming specialists, it simply didn’t seem worthwhile to them to upskill into a neighboring sector even though Crimson Permanent Assurance was eager to facilitate that shift. These employees saw it for what it was: treading water. With a team this highly educated, a lack of skills wasn’t their problem; it was a lack of adaptability.
...with cognitive automation the half-life of hot skills is short and getting shorter by the day.
Still, a skill-centered view of human capital is a hard habit to break. The hot skills tool was technically interesting, but I rather bluntly told its lanky creator, “What are you going to do in two years when the hot skills inevitably shift?” Upskilling doesn’t result in long-term positive outcomes for employees or employers because, with cognitive automation the half-life of hot skills is short and getting shorter by the day90. There is no routine skill or knowledge that is truly robot-proof.
As we saw with Herr. Bubba, retraining high-skill workers into new fields fails when their whole career has been spent in the service economy. Just like most doctors, recruiters, teachers, and the rest of us, their careers have not prepared them to explore the unknown. As we’ve seen, even when given the bait instead of the switch, most people fail to leverage creative complementarity. Policy papers covering the future of work tend to treat humans as endlessly fungible widgets—we’re cars: if demand for SUVs rises, simply retool a sedan factory to produce more SUVs91. But people are not widgets, and the increasing demand for creative jobs in Fig. 4 won’t funge them. “Retooling” us requires decades of life experience and a much grander investment. Absent deeper intervention, few can enter the creative economy just because the opportunity is in front of them.
Similar promises about globalization producing new knowledge economy jobs for coal miners and bus drivers have failed to materialize92. Whether we look at physical or cognitive automation, offshoring or outsourcing, labor substitution drives a massive dissolution of the developed world’s middle class. Unfortunately for them, they’ve been outvoted and their share of the economy divided up amongst the winners. The developing world has cast their vote for globalization–its new middle class funded by its share of the spoils93. Consumers have cast their vote for anything that delivers lower prices, paying for it with their share of the spoils. The final vote put the remaining spoils into the treasure chests of the companies themselves, their senior management, and principal equity holders94.
The impact of globalisation has important lessons for cognitive automation. Its promise was real…is real, and parts of that promise–lower prices and a massive reduction in global poverty–were kept. But it was all predicated on those promised new jobs, the lazy myth of the Industrial Revolution. The demand for creative labor in developed economies has certainly grown, but the people who lost jobs need not apply. In one interview on NPR prior to the 2016 US presidential election, an out of work coal miner complained, “For 10 years they’ve been promising retraining and new jobs. I just want my old mining job back. At least Trump said he’d try.” The violation of that promise clearly has had meaningful political repercussions: political polarization, nationalism, and racism95.
Stormy Waters
In addition to the challenges Crimson Permanent Assurance was facing in upskilling to head off redundancies, internal research revealed employees burning out or not feeling they had any control in their careers. This very hierarchical company exerted constant pressure for junior employees to “move up or move out”. We began collaborating on a system to address these two sometimes conflicting needs: giving employees control over their careers while guiding long-term career trajectories that won’t steer them off the edge of the economy. We drew on masses of Crimson’s employee data and modeled career paths within a latent, hyper-dimensional space of projects, mentors, industries, educations, and yes, even skills.
Think of this “latent space” as a very complex map of career trajectories. The algorithm produced the maps by searching for manifolds in this high-dimensional space that produced the smoothest trajectories through this wild sea of jobs. The result was something like a hurricane forecasting map, but in place of storms it tracked individual careers. An employee could see the trace of their career tracked through the past along with those of other employees. When that trace reached the present day, it transformed from a single line into an expanding confidence interval of possible futures, like the uncertainty of a hurricane landfall, representing the distribution of career outcomes from others in the past. The majority of employees followed four broad trajectories: some churned out; others had modest careers; many became creative contributors; and a rare few reached leadership roles. We focused on understanding how employee choice at key moments contributed to different outcomes.
Rather than gut checking themselves on a big career decision, we gave them a tool that revealed the outcomes of those who had followed similar paths to similar decision points.
We identified several explicit events and choices for Crimson employees that were points of highest uncertainty, moments where career trajectories diverged suddenly toward differing outcomes. On the map, careers would largely track together until these key moments where many would suddenly break away to follow different paths. We used this discovery to create a career forecasting tool for junior employees as they approached these key moments. Rather than gut checking themselves on a big career decision, we gave them a tool that revealed the outcomes of those who had followed similar paths to similar decision points. Our model clustered all of those past employees into various outcome archetypes and revealed the patterns of choices made by each archetype. Which training program produced the most engineering leaders? What type of mentor best sponsored growth in design? Which project assignment might take me furthest?
(Along with the four broad career trajectory patterns, we found a fifth pattern that kept popping up: no pattern at all. These employees looked like crazed, chaotic zigzags on the map. The only thing that they had in common with each other, beyond not looking like anyone else, was that all of them either churned out quickly or went on to high-level leadership roles. While the majority of leaders followed well-worn paths to success, it was interesting to see that the people that didn’t fit any career model at all were the ones that ended up being some of the highest value creators…if they managed to avoid falling off the map entirely.)
Building tools that help people is immensely satisfying, whether a diabetes AI for my son or big data tools for career decisions. Scientific curiosity makes the day-to-day work easy, but evidence that it made a difference to someone makes the work meaningful. I’m proud of our career forecasting collaboration for its insights and practicality, but we knew the tool was fundamentally limited. It still represented employees as “keywords on a resume” rather than as the factors that define a real person. During the course of the project I consistently pushed for a focus on the deeper factors that drive long-term success, but it was frustratingly difficult to break out of deeply ingrained HR practices96. For many, not just at Crimson, I’m a mad scientist proposing the absurd. It’s so much easier to solve the “obvious” problems piling up in your email every week97, like an uptick in demand for SAP skills paired with Energy industry experience.
The Crimson Permanent Assurance Company had every reason to just solve easy problems. Though they forecasted lay-offs approaching for half their employees, it wasn't because business was bad (business was great!). They simply didn’t need people that were only capable of doing what they were told. Like so many other believers in the lazy myth of the Industrial Revolution, they had assumed creative complementarity would just emerge to meet demand, but substitution was already spreading within Crimson’s walls. Despite genuine effort, projects to put long-term value back into their employees were largely failing. So, the swashbucklers of the Crimson decided they needed a little mad science in their lives. But to tell the story of Project Albatros, we’ll first need a tour through stories of fanatics, marshmallows, and horticultural sacrifice.
As for my loan application, AI can run the financial models faster, cheaper and more accurately than any human. Without the human in the loop my application was approved immediately. Yet, AI is far from a perfect oracle of financial futures98, and despite the bait-and-switch–“Automation allows lenders and banks to focus more on the customer experience and less on comparing data from various standardized forms”–I have no meaningful or personal relationship with my bank. The only true enhancement of my customer experience is that it was all handled in a single trip to my toilet.
The truth is that most companies and “thought leaders” are simply disingenuous when it comes to the future of work. One of the cultural paradoxes of the Technology Industry is that it tells itself and the world that it’s building tools to democratize labor99, while Tech itself only hires “A-players”. As we’ll see in the next section, Tech has learned to stop worrying and love the chasm.
The collected works of Prof. Schlashenschlessen
“Why do all ambitious women have flat heads?”
– Dame Stephanie “Steve” Shirley





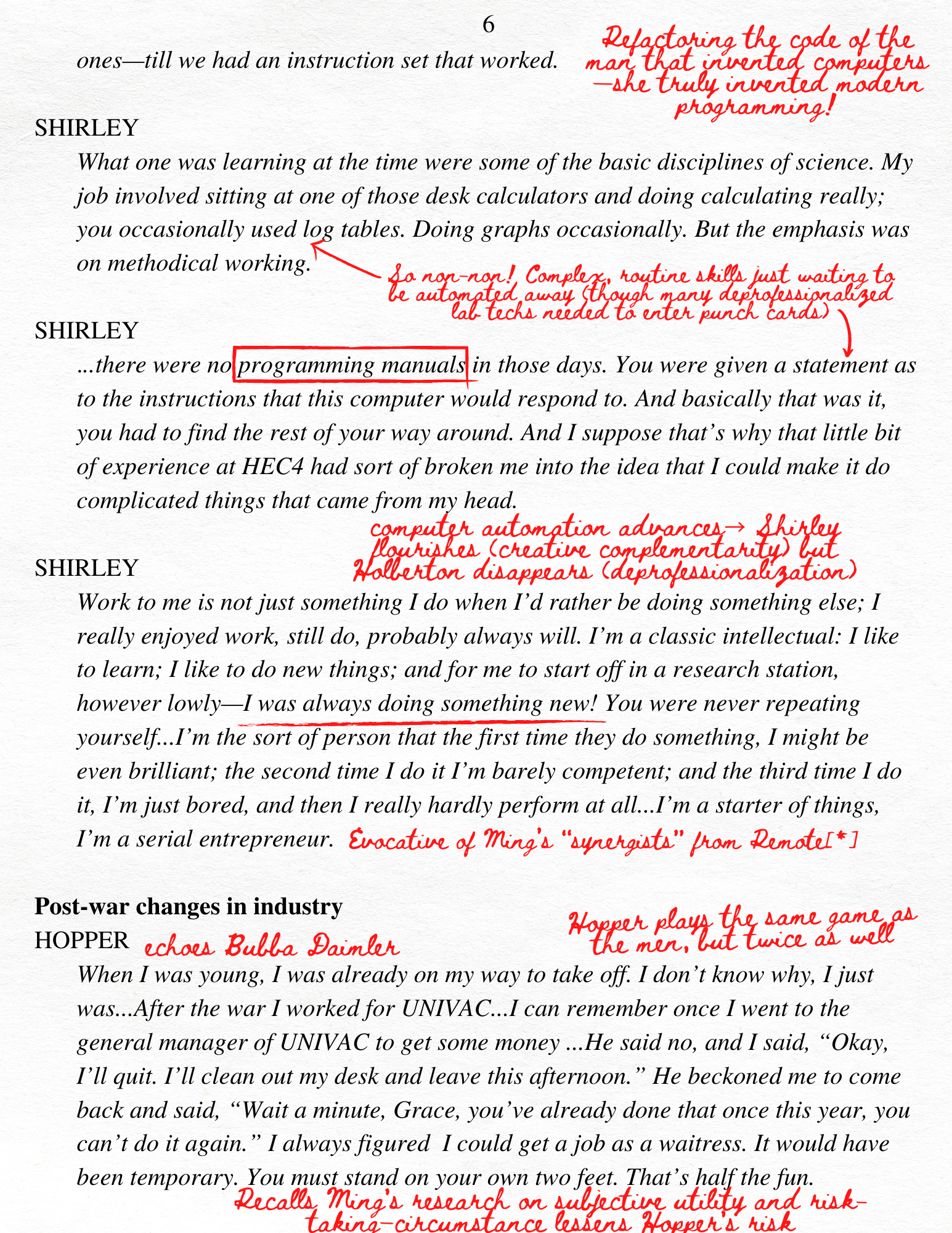
*







