Innovating Innovation
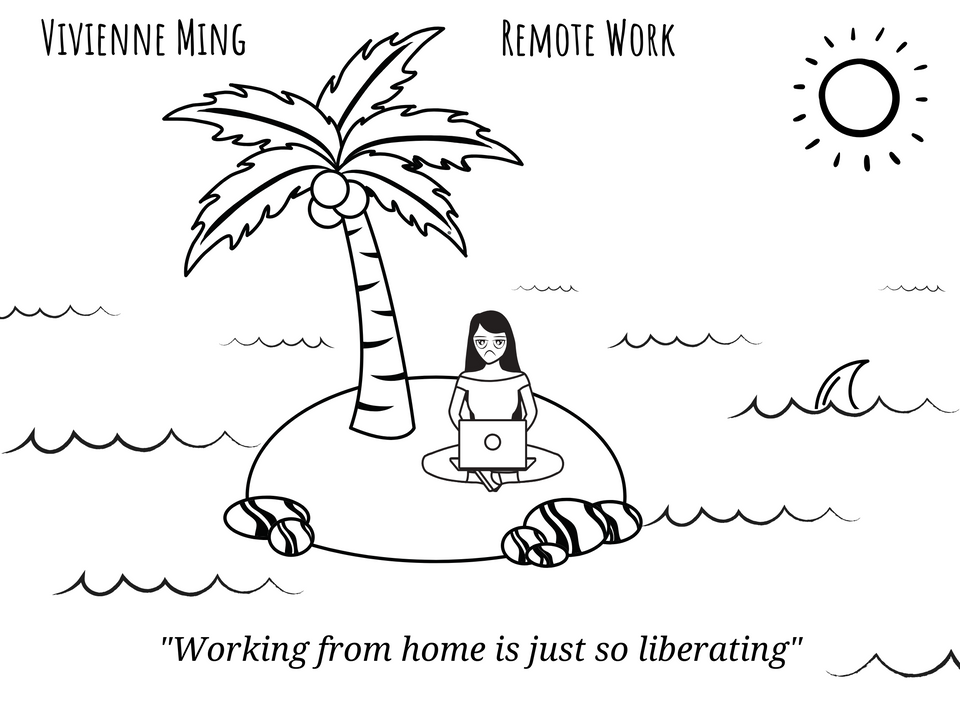
Distributed Innovation
“The meeting of two personalities is like the contact of two chemical substances: if there is any reaction, both are transformed.” Jung’s simile is perhaps my all time favorite metaphor. On its surface, Brownian people drift through life, occasionally colliding and, by chance, transform each other. Jung’s interpersonal chemistry captures two of the greatest worries about remote work: without those chance collisions what drives innovation, and without interpersonal transformation how do we increase inclusion? Over the last year, I’ve been asked these two questions about remote work more than any other23.
If innovation is just an accumulation of randomly colliding ideas and personalities, as so many assume, all we can do is bring more and more people together, hoping to scale up the collisions. Density brings serendipity. Innovation requires bigger cities, more universities, in-person conferences, and a seemingly absurd amount of time spent lingering around the watercooler waiting for just the right off-hand comment to spark an “ah-ha” moment.
It’s not an unreasonable metaphor. We already know that research, innovation, and industry are concentrated disproportionately in the largest cities. Just ten US cities account for 48% of its patents and 33% of its GDP, even though they make up less than a quarter of the population. Across science and industry, as activities increase in complexity, they also increase in spatial concentration. Some have even argued that a single parameter, population, accounts for all productivity and innovation variation across cities24. If spatial concentration and pure serendipity are crucial to innovation, then “remote innovation” might quickly displace “army intelligence” atop the oxymoron rankings.
Insights from distributed computing don’t offer much hope. In these distributed systems, all communication is passed through narrow pipes, requiring potentially complex (de)serialization algorithms and long latencies. When all work passes through these same pipes, the sort of scale-dependent serendipity assumed to drive innovation is nearly impossible. Virtual watercoolers lose the chance interactions of the real thing, as everyone is there for the explicit purpose of socializing. Company-wide open blogs and social channels are popular remedies for isolation, but as we’ve noted earlier, winner-take-all effects in large-scale networks can easily reduce diversity of thought rather than promote innovation.
If we’re just particles waiting for a chance reaction25 through rate-limiting pipes, then it’s hardly surprising the big, complex projects at Baidu ground to a halt when the world went remote. Worse still, all of this is within the broader decline in VC-backed early-stage startups and corporate innovation common during recessions. Capital flows away from the truly novel to sure up more mature bets.
Fortunately, Jung’s metaphor has a deeper mapping between the complexities of chemical reactions and interpersonal relationships. Imagine the sophistication of advanced chemical engineering, or even coronavirus vaccine development, reduced to the brutish simplicity of two enormous vats of chemicals dumped together in a tub. No catalysts or buffering agents. No stoichiometry. No knowledge of the underlying chemistry at all. Just swirling molecules at massive scale.
While the interaction of any two specific molecules may be a fundamentally probabilistic process, modern chemistry grants detailed control of its product by biasing the statistics towards the outcomes we desire. Perhaps the pipes carrying distributed work can play the role of pipettes carrying chemicals and transform innovation itself.
Innovating on Innovation
Despite the proliferation of “me too” startup incubators and dubious corporate innovation handbooks, no “innovation engineering” fuels creativity the way chemical engineering fuels Pfizer, Dow, and Chevron. That doesn’t mean we know nothing. For decades, researchers have been quantifying the neuroscience, psychology, and social dynamics of innovation. For example, we’ve identified the complex and effortful interplay of brain networks that drive our capacity to generate original ideas, and have even identified the brain regions behind high-quality jazz improvisation. We’ve identified the subtle interplay of attention, memory, and reward that leads to those almost mythical ah-ha!‐moments, although it seems eureka is much less bolt-of-lightning than working memory and fluid reasoning31. And the brains of Nobel prize winners in chemistry and literature look very similar in moments of creativity. Perhaps it’s not so surprising, then, that Nobel prize winning scientists are three times as likely as the general public to pursue artistic interests outside the lab, but importantly, their art and their science complement each other, reflecting the same underlying passions.
Most crucial to remote work, distributed cognition research has revealed much more about collaborative innovation than just smashing people together. Consistent with the basic idea that density drives collaborative innovation, two labs that are close to each other on university campuses are 3.5 times as likely to collaborate, but the story immediately becomes more complex. For example, once a collaboration has begun, separation doesn’t disrupt the relationship. In fact, separation improves the quality of research outcomes by promoting more independent “research trajectories”. The original relationship established by colocation is the catalyst for collaborative innovation, but without a rate limiting reagent to prevent cognitive homogenization, the value of that relationship reaches premature equilibrium with little more to be gained27.
The research on how colocation affects innovation reveals a much more complex story than density=serendipity. While colocated labs are much more likely to collaborate, the novelty and quality of the projects they produce drop off. This tradeoff clearly indicates that while we want people to interact, simply pouring everyone into the same vat is as brutish and inefficient as one might imagine. Rather than an undifferentiated equilibrium, our goal for innovation must be dynamic allostasis. The pipes connecting us together can be an advantage, titrating our interactions to optimize the tradeoff.
For example, the flow of knowledge carried by business travelers from one city causally changes the growth in economic activity in a destination city. This finding shows that if you change those travel networks–snipping a connection here, adding another there–you change the city itself. Innovation and economic activity within big cities are about much more than size or density. Like personalities, the mixing of cities leaves both transformed.
Optimizing innovation is not just a matter of how we structure people but how we structure knowledge and information. Searching for books, articles, and websites would be nearly impossible without some kind of hierarchical information structure. Imagine walking into a library28 where the books are simply strewn around the floor with no structure–no French literature section or “Children’s Corner”. You have to kick Madame Bovary out of the way to find The Lorax. But if you’re not trying to find a specific book, if what you want is a novel idea, then too much structure also holds you back. When information is presented in a flat, unorganized structure it promotes cognitive flexibility, improving creative problem solving. Flat information structures even helped individuals come up with more creative career ideas.
There is a parallel between research on knowledge structures and spatial structures in promoting innovation. On their surface, both fundamentally rely on dumb luck powered by masses of unstructured interaction. But just as titrating interactions between collaborators might maximize innovation, dynamic information structures might act as catalysts for new insights. For example, participants were more likely to develop useful insights in creative problem solving experiments when parallels to seemingly unrelated problems were made salient.
Another set of experiments in complex problem solving showed that storing subjects’ best past solutions for speedy retrieval increased mean performance but decreased exploration. Fascinatingly, this research showed that ubiquitous access to past ideas had the same effect as unrestricted social influence. When people are allowed to interact without restriction while exploring complex problems, the average problem-solving performance of the group increases. Hidden by this average performance increase, however, is a drop in the quality of the best ideas. Satisficing innovations29 both crowd out better, later innovations and preempt continued exploration. The researchers found that the best solutions emerged when a period of separation preceded social interaction. In both knowledge structures and social networks, something as simple as making access intermittent can act as a rate limiting reagent, improving average performance while maintaining the best solutions.
The structure of interaction within a team might even be more important than interactions across a network. A study of collaboration within academic labs reveals the crucial importance of the internal social architecture in tightly connected teams. The researchers were able to leverage data from the asynchronous tool Dropbox to track interactions between collaborators in fine detail. They found that elite teams tended to be small, possibly counteracting the homogenizing effect of large groups. They had flat hierarchies, with group members contributing to projects more equitably. While their contributions were equitable, the best teams also evidenced complimentary diversity: individuals’ contributions reflected their relative expertise. But even elite teams weren’t perfect. The research found that elite labs were more likely than others to collaborate based on preexisting relationships. In following the path of least resistance, elite labs fail to capture the innovation capacity of novel collaborations.
In 2012, Google launched Project Aristotle to understand why certain teams were more successful than others. On the surface, highly successful teams could be wildly different, but some teams leveraged those differences in powerful ways that others could not. Strong norms within a team seemed to be a crucial factor for success if those norms supported two specific qualities. First, just as with the Dropbox study, elite teams had flatter hierarchies with more equitable and complementary contributions from individual members. In an echo of a large body of research on creative problem solving, when a lone member dominated, collective intelligence dropped. Second, elite teams demonstrated strong average perspective taking. Strong norms promoting prosocial perspective taking in turn promote psychological safety, a form of interpersonal trust that rewards constructive risk-taking. In the absence of psychological safety, individual members withhold their contributions and the quality of both the average and best solutions drop. Psychological safety buffers teams from large variations in personalities and identity, turning an acid comment into a creative catalyst.
We’ve only touched on a tiny portion of the vast research literature on innovation and creative problem solving, and yet already patterns emerge. There is a microstructure within individual innovation cells (e.g., flat hierarchies within teams). These individual cells interact through a macrostructure of inter-team collaborations and information flows. For innovation engineering to emerge from the existing science, we must grow our understanding of the macrostructure beyond the passive world of density=serendipity. Those narrow pipes can give us fine control of the social graph of innovation, but what do we do with them? One candidate model of innovation engineering comes from the Defense Advanced Research Projects Agency (DARPA).
Like all major US funding agencies (e.g., NIH and NSF), DARPA exists to promote research and innovation principally by controlling the purse strings of science. Unlike its peer institutions, DARPA is both small and flat. The individual DARPA program managers (PMs) do more than decide who gets funded. They leverage an unusual amount of flexibility and autonomy to drive innovation within a specific domain by bringing together disparate cells, whether academic teams or industry labs, for workshops and collaborations. In essence, they are innovation matchmakers, peeking in on the activities of individual cells and strategically manipulating connections between them. In a review of organizational innovation, a group of researchers identify the existence of “idea scouts” and “idea connectors”30 that have a disproportionate impact on innovation; in many ways, the DARPA PM formalizes both of these roles in a single individual that combines the technical insight of scouts and the social engineering of idea connectors.
The autonomy of DARPA PMs creates space between funding oversight and risky projects. They de-risk the work of individual cells, allowing them to explore ideas that wouldn’t normally get funded. Of course, that also means that many projects “fail”, but the matchmaker PM can share the insight and learning from those failures via their network. In this way, the PMs themselves replace density=serendipity as the macrostructure of innovation, connecting the microstructure of individual cells to capture the full capacity of the system.
The distinction between macro- and microstructure takes Jung’s metaphor far from a vat of undifferentiated chemicals and into the realm of biochemistry, where “inside/outside” dominates over density. Membranes allow cells to control their interaction across the intercellular matrix while preserving the integrity of their internal processes. Individual cells are able to interact, but those interactions are modulated by their membranes. While narrow pipes are a drag on so much of distributed work, in innovation engineering they can be transmembrane protein, ion channel, and extracellular matrix all rolled into one.
Remote innovation might seem like an oxymoron, but in fact distributed work might be the crucial catalyst for innovation engineering to finally emerge. We know its microstructure: flat, small, equitable cells leveraging complimentary diversity and psychological safety. We begin to understand its macrostructure: allostatic tensions and finely tuned networks. Distributed work gives us both unique insight into the microstructure and unique control of the macrostructure.
Innovation Engineering
So, let’s invent innovation engineering. Fortunately, we’re not starting from scratch. At Socos Labs, I’ve been exploring these ideas for years, long before Covid-19 forced us all to work from home. Our innovation engineering framework incorporates tools to analyze the microstructure dynamics of ideas and personalities within each cell and mechanisms to control the macrostructure of collaboration and knowledge dissemination between cells. The basic framework is illustrated in Figure 1. It begins with incubation, where individuals cycle between isolation and collaboration within their cell. Next is a novel but crucial process of maturation, in which innovation cells collaborate together in small clusters to refine an idea into a robust practice. Finally, clusters engage in strategic dissemination to establish new practices across the community.

At the center of this framework is the matchmaker, a new role inside organizations modeled on DARPA’s PMs and “idea scouts”. In the following section, we’ll describe how well-trained matchmakers track incubation of novel ideas and dynamically titrate the connections between individuals, cells, and clusters to accelerate innovation. Our illustration of this process uses examples of machine learning tools that augment matchmaker capabilities, but Socos’s framework isn’t contingent on technology–matchmakers should be able to carry out these steps independent of any specific tool31.
Matchmakers peek inside each cell’s microstructure to observe the internal dynamics of creation and communication. While this occasionally means joining in on synchronous conversations32, asynchronous tools give matchmakers access to the state of innovation within and across cells. Socos Labs and others have developed tools to help matchmakers manage the allostatic balance between individual and collaborative ideation. Given the importance of interpersonal dynamics to innovation (e.g., complimentary diversity), our framework leverages personality modeling, critical for the mixing and remixing of collaborators. We’ve also developed models of the conceptual space of new ideas, giving matchmakers greater insight into the landscape of innovation. Finally, our framework models the temporal dynamics of innovation, allowing matchmakers to form new connections between cells and ideas at just the right moment, or even slow the flow of intercell communication when novelty is being drowned out.
Figure 2 captures some of the dynamics of the incubation process within cells (broad purple circles). In the figure, the matchmaker is shown outside of any cell but directly monitoring activity across asynchronous tools and knowledge resources. A cell of six densely connected individuals is shown collaborating around a central asynchronous document, forming a supermind. Individually, they may be engaged in isolated ideation (person 1), in synchronous conversation (4, 5, and 6), or in information gathering outside the cell, either with knowledge resources (2) or other people (4).
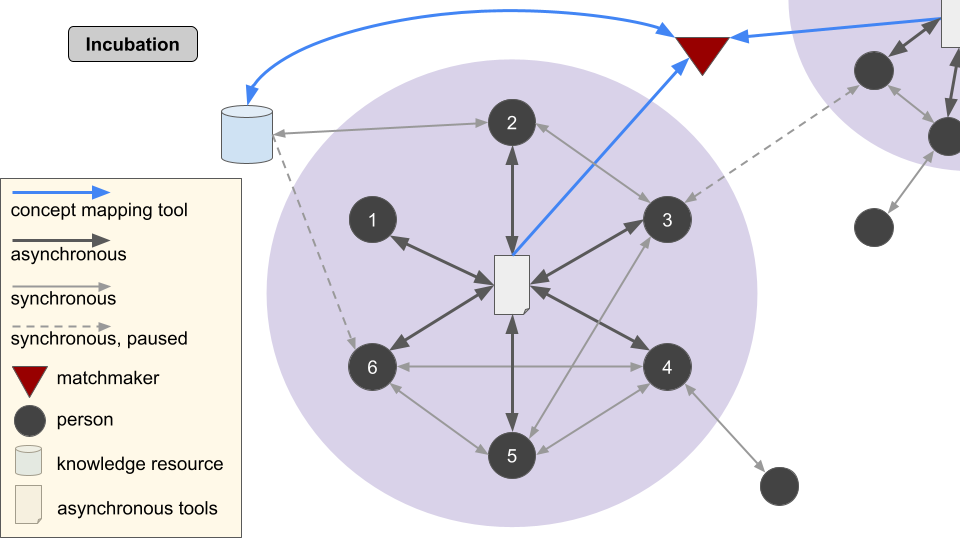
These six individuals aren’t brought together arbitrarily. Some might be long-term collaborators, while others are joining for the first time to bring unique expertise or perspectives. Matchmakers and project managers work together to design complimentary diversity into cells, exploring mixtures of personalities that maximize innovation. Personality modeling has been used to recommend research collaborations, predict team performance, and study peer-to-peer interactions in education. My first experience in this domain was a project to identify beneficial student cohorts in massive online courses. Unsurprisingly given everything we’ve learned, dumping 30,000 students into an unstructured discussion forum is not a recipe for success. Grouping students into smaller cohorts of 5-10 peers with their own subnetwork improved course outcomes compared to students without a cohort. But we also found some of the first evidence of complimentary diversity, strong indications that a successful cohort needed the right mix of backgrounds, personalities, and experiences. There wasn’t a single pattern for success, but active cohort matching improved outcomes33 by 34% above random cohorts alone.
In a completely different domain, I developed an algorithm to recommend novel connections at professional conferences. The algorithm worked by analyzing the personal and social profiles of pairs of individuals (dyads). Importantly, we discovered that the most successful connections were not defined by similarity alone (homophily). While most people shared touchpoints of similarity, likely facilitating psychological safety, relationship patterns across dyads were predominantly about differences, but those differences weren’t arbitrary–everyone had patterns. For example, one pattern emerged that actually applied to me. We might call it the “geeks hang with nerds” pattern: while I’m a highfalutin scientist that’s rather snooty about my science fiction and doesn’t fully understand why anyone would attend Comic Con, a dominant pattern in my social network are Comic Con fangirl engineers with an appreciation for the campy.
Based on these past projects, our framework models personalities using a combination of explicit and implicit measures (e.g., smart survey methods and language modeling across company communications)34. The insights derived from this system are crucial for maintaining the critical allostatic tension between trust and novelty. As we’ve described above, as cells work together over time, the novelty and impact of their innovations decrease. Our framework gives matchmakers the ability to subtly mix and remix collaborations, keeping them at their critical point. This remixing infuses existing cells with both new knowledge and new interpersonal dynamics, but it depends on matchmakers identifying the right pairing. (As we will discuss in the next section, there is a paradoxical tension at the heart of successful innovation collaborations that all organizations must overcome.)
While personalities are crucial, innovations are the actual product. Innovative ideas can be visualized as a dynamic map of concepts, with neighboring ideas having greater influence on each other than those further away. In our framework, matchmakers can use these maps to nudge apart cells to prevent herding around obvious solutions and connect seemingly disparate ideas to uncover novel synergies. Our tool emerged out of an internal project, Cognitive Overflow, which I developed years ago to help map my labyrinthine library of research notes. I would frequently find myself writing on a subject with vague memories of a relevant research article or previous project. After an hour of fruitless keyword searches in my notes or online, I’d have nothing to show for the effort but tears and curses. Cognitive Overflow created a personalized conceptual map of my library. As I wrote, it pulled up relevant research that I could accept or reject without ever running a search. I could even use Cognitive Overflow to remap my library to reflect other people’s conceptual maps and uncover novel connections that might not have occurred to me naturally.
In our framework, this tool can be used within cells to accelerate knowledge discovery beyond either flat or static knowledge structures; however, it plays a distinct role for matchmakers. By applying similar concept maps to the evolving content captured by asynchronous tools35 (blue lines in Fig. 2), matchmakers can track and even guide the dynamics of idea creation. They can nudge individuals away from less productive concept spaces or from herding around low-risk ideas, spreading bets and encouraging greater exploration36. In Figure 2, the matchmaker is nudging person 2 with a novel knowledge structure (like Cognitive Overflow’s map sharing) to make underexplored concepts more salient. Access can also be paused, as with person 6, to prevent exposure to satisficing solutions that might head off future exploration. Even more powerful nudges come from reconfiguring the social connections between cells, such as how the matchmaker has paused the social connection between person 3 and an individual in a neighboring cell.
Crucially, matchmakers’ nudges must come at just the right time. For example, an extensive research literature has identified the importance of incubation periods for innovation. Neuroscience has revealed that incubation periods involve unconscious processing of the problem rather than mere distractions or interruptions. Cells that engage in independent work before group collaboration generate more ideas of higher quality and come away with a deeper understanding of the problem. In fact, if matchmakers bring collaborators together too soon, the number and quality of new ideas decreases.
I had my own rather exaggerated version of this experience during my first year of graduate school. I had “invented” a novel algorithm for decomposing sounds and images into a highly efficient “dictionaries” of “code words”37. I had convergence proofs and everything. After writing a couple papers and presenting this as my first year project, my research advisor said, “You might want to read this,” and handed me Stephen Mallat’s paper on matching pursuit. It turns out that I had re-invented an algorithm that had already been invented by the guy that had literally written the book on wavelets38. I could have felt bad about discovering that I hadn’t discovered anything39, but in fact my understanding of the material and its application to the brain was greatly enhanced by all the work that I had done on my own. When I later learned that the algorithm was known by mathematicians as von Neumann’s method, I decided to just be proud that I had the same idea as a man who’s in the running for smartest person of all time. And as it turns out, I was able to stand on the shoulders of these giants and convert the algorithm to a truly novel form of machine learning that could learn the optimal dictionary directly from the sounds and images themselves.
I’ve always wondered whether my advisor intentionally withheld Mallat’s research to spur my own creativity or if he just didn’t see the connection until we started writing our paper40. Whether intentional or not, he essentially played the role of matchmaker, blocking a macroscale connection which would have prevented incubation of my own ideas. If I’d had the “answer” from the textbook I would never have fully explored the concepts that led to my breakthrough. Research supports this seemingly paradoxical intuition: slowing down the process of knowledge diffusion in dynamic networks actually speeds up innovation. Further, innovation spreads more slowly through densely-connected networks, like a company's social channels, and while highly efficient networks can quickly identify satisficing solutions to complex problems, collective intelligence (long-run innovation) suffers. Both poorly connected and densely connected communities perform worse than moderately connected. This inverse relationship between knowledge diffusion and innovation diffusion may seem confusing if you assume an innovation is just a piece of knowledge. Instead, these findings illuminate a profound distinction: knowledge is information; innovation is practice.
I have a long and colorful history of inventing broccoli. I have detailed analyses on its nutritional value and extensive experiments proving its health effects. Every time, I’m convinced my broccoli will revolutionize the market and people will beg to buy it. On sales calls, customers love the idea of broccoli–they’re blown away by all of my scientific research on this weird, green, alien-looking product, but then they say, “So...what the hell am I supposed to do with it?”
My potential customers tell me they’re committed to their brownies. The issue isn’t just that the brownies taste better; brownies have established distribution channels, tax incentives, and best practices. They “get” brownies.
They believe my research about broccoli, but nobody has any experience with it actually improving their lives. It all seems so abstract. Then they take a bite of raw broccoli, and I can see that the sale is lost. Neither they nor I have any recipes for broccoli. I assumed that the idea of broccoli, with all of my scientific evidence, would be enough to spark my customers’ creativity, but nobody is willing to put in the effort to come up with their own recipe just so they can buy my product. Everybody loves the idea of broccoli, but nobody wants to buy it41.
Over the years, I’ve invented broccoli across numerous industries and scientific disciplines. Again and again I’ve looked on as people have said, “This is the most amazing thing I’ve ever seen, but what the hell am I supposed to do with it?” My new ideas, even fully developed new technologies, were not a practice. New ideas require new behaviors and affordances to become a practice. The cost of turning new ideas into practices and the uncertainty that the effort will pay off suppress adoption of potential innovations. The challenges of disseminating innovation throughout a community are explained in greater detail below, but in our framework, a maturation phase is needed first to overcome these barriers and turn ideas into mature practices. Where ideation involves microscale dynamics between individuals and their cells, maturation involves macroscale dynamics between cells and cell clusters as shown in Figure 3.
Where an innovation cell is a densely connected set of individuals exploring a problem, cell clusters, as illustrated in Figure 3, are small subgroupings of cells within a broader community that collaborate on maturing a new innovation from knowledge into practice. Cells within a cluster might share domain interests, areas of expertise, and interpersonal connections (thick blue lines), but they must possess the same kind of complimentary diversity as individuals within a cell. Research on large organizations shows that when subgroups are semi-isolated from the broader community it prevents cognitive homogenization and herding around satisficing solutions, maximizing “equilibrium performance” of collective intelligence. Rather than rely on the paradoxical serendipity of moderate connectivity, matchmakers dynamically titrate connections within a cluster based on the incubation cycles of the individual cells. This dynamic moderation leads to “the highest equilibrium performance” of collective intelligence.
Where the received wisdom of density=serendipity is inefficient but functional when the spatial constraints of an office slow information flow, in distributed work it can be as crippling as no connections at all. The role of the matchmaker is to maintain optimal allostatic balance by removing and creating connections at just the right time. While it might seem challenging for matchmakers to intervene on a complex network, there are many examples of success. In neuroscience, groups are developing control strategies to target seizure suppression in the dynamic brain networks of epilepsy patients42. Back in the domain of social networks, analysis of temporal dynamics can predict social behavior with high precision or predict students' effort and performance from the social dynamics in a class. And research on innovation itself shows that matchmakers can actively induce intermittent interactions between cells that lift the novelty and impact of the best ideas. Informed by the microstructure within cells, matchmakers can strategically regulate cross-cell and cross-individual interaction. Titrating interactions maximizes the trade-off between independent thought and creative collaboration, preserving the average quality of work while lifting adoption of the best solutions.
Figure 3 traces the process of maturation. At Step 1, all of the cells are engaged in the early stages of ideation. There are various pre-existing social connections between individuals in different cells (dotted blue lines), but the matchmaker has paused direct communication. At Step 2, Cell A has developed a potential innovation, and maturation begins as the matchmaker opens up lines of communication in Step 3. Remember, at this point Cell A has invented broccoli. They have an exciting idea which is not yet a practice, and as we noted above, people resist investing in raw ideas. So, not only is this investment the cluster’s explicit responsibility, but the matchmaker leverages social engineering to stack the deck in its favor. The specific connections activated by the matchmaker are not chosen at random, but rather, selected to maximize uptake by leveraging either high-trust past working relationships (blue lines) or strong, novel personality matches (green lines). The goal of the matchmaker is to connect people that “get” each other, driving engagement with the raw idea. Even as the connections between Cell A and its neighbors are activated, the connections between Cell B and Cell C remain paused, allowing them to independently incubate the incoming discovery.

In Step 4, Cells B and C have incubated their own variations of the original discovery. The diversity within the cluster allows it to identify new applications and hidden challenges associated with the new idea. At this point, the matchmaker opens all channels of communications between cells (Step 5), allowing the entire cluster to find an equilibrium in a set of behaviors and affordances which generalize across every cell. By Step 6, the cluster has matured Cell A’s original idea into a robust practice ready for dissemination throughout the community.
In other words, Cell A invented broccoli. Cell B, noting the nutritional value when raw, developed a recipe for juicing it. Independently, Cell C came up with a reasonably tasty recipe for sauteed broccoli. Cell A noted that they don’t have a juicer and that broccoli juice sounds pretty disgusting anyhow. Cell B argued that juicing it is the best way to preserve its nutrients, and so Cell C began exploring the optimal sauteeing time to maximize flavor while maintaining nutrition. Cells A and B, both with saute pans of their own, begin testing salt and garlic in their recipes. In the end, the cluster arrived at a tasty and healthy recipe requiring only tools and ingredients available to everyone. The idea of broccoli is now a practice of sauteed broccoli with garlic.
With a mature practice, a cluster can now disseminate a true innovation throughout the community. Just as with ideation and maturation, optimal dissemination relies on moderate levels of connectivity and dynamic allostasis. This emerges, if rarely, even in the density=serendipity model. For example, the new social connections that result from individuals moving from high-impact smaller teams to larger scaling teams account for much of the global gain in innovation. Our framework turns this passive serendipity into intentional design43. The matchmaker actively engineers the macroscale dynamics by both remixing cell membership and modulating connections. One of their goals is to create “non-redundant connections” between cells, which play a crucial role in increasing adoption of innovations.
Successful dissemination is represented in Figure 4, Panel A. Initially, the matchmaker (lurking disreputably in its shady crimson triangle) has paused all of the controlled communication channels between clusters44. This allows individual cells and clusters to independently cycle through phases of incubation and maturation. In Steps 1–4, the central cluster develops a new idea, reaches equilibrium, and is ready to disseminate an innovation to the community. In Step 5, the matchmaker turns on communication only between the central cluster and its closest connections. With all of the other connections still paused, the central cluster can leverage trust and established relationships to reduce adoption uncertainty, and its three cells can leverage their non-redundant relationships to further drive adoption. As new cells adopt the innovation, the matchmaker strategically opens additional communications channels, creating a synchronized wave of transmission throughout the network (Step 8), which drives it towards equilibrium (Step 9).
Without the maturation phase or the intervention of the matchmaker, networks suppress innovation through both winner-take-all effects and high adoption thresholds. In Panels B and C, we see the same network of innovation cells attempting to develop and disseminate new ideas within a standard density=serendipity model. In Panel B, the initial cell has a novel idea which is immediately communicated to its neighbors without time for maturation. Without an established practice, its neighbors in turn either ignore the idea or come up with their own variations on it. With no variation able to reach equilibrium, the existing practice (purple cells) eventually drives out the new ideas.
In Panel C, the original cell managed to develop a fully formed practice on its own. It immediately begins sharing this practice with others. Although they are likely to adopt the fully formed innovation, this communication lacks the rich, non-redundant set of channels that the central cluster would have brought to dissemination. With most existing relationships still committed to the initial practice, the originating cell can’t generate the synchronized wave of transmission needed to maximize adoption.
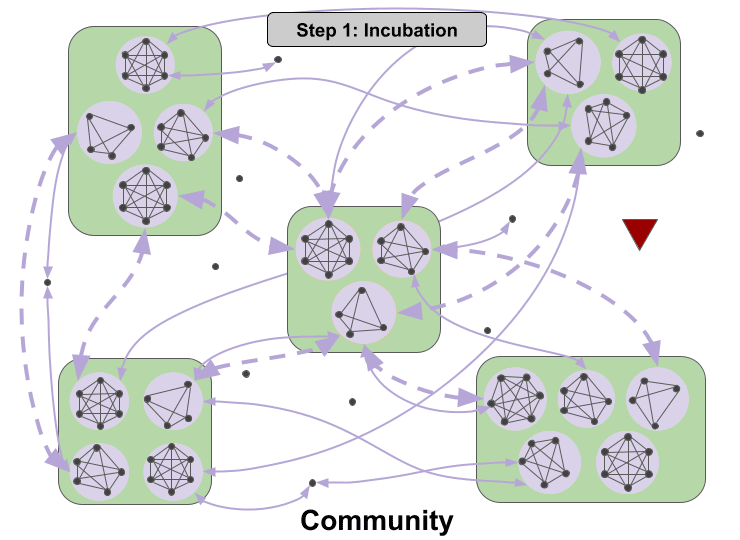



Socos’s framework for innovation engineering introduces a number of crucial advances: tools that augment matchmaker interventions; the remixing of personalities and relationships throughout a community; maturation of ideas into practices; clusters designed to leverage trust; and, matchmakers that peek in and orchestrate it all45. These innovations on innovation help to lift the rate of invention and dramatically increase the adoption of novel practices. All of this leaves one last question: how do you incentivize individuals and cells to maximize the collective intelligence of your organization?
Perhaps the most obvious starting point is a marketplace for innovation. Acemoglu and colleagues have posed innovation as a “resource allocation problem” where communities are confronted with aligning expertise and preferences of individuals and cells with the often hidden needs and complexities of unexplored problems. The idea can be thought of as a prediction market in which “dynamic pricing mechanisms...induce workers to self-select” into different problems that maximize their returns. If innovation were purely a skill-matching problem, we would expect market-based methods like this to maximize collective intelligence (defined here as the number of mature innovations in full practice).
Unfortunately, open markets seem to quickly settle on satisficing solutions rather than substantive innovations. While this phenomenon is driven in part by densely connected networks and (paradoxically) easy access to information, it turns out that in innovation, market-based incentives “produce herding effects, reduce information available to the group, and restrain collective intelligence.” Each individual maximizes their returns, not by coming up with revolutionary ideas, but by letting the majority de-risk their choices.
If you are feeling bad for Adam Smith, you might be happy to know that you can do much worse than an innovation market. A simple reward scheme in which individuals or cells receive fixed rewards whenever they develop a new idea substantially reduces collective intelligence compared to market incentives. But one incentive structure dramatically outperforms them all: “minority opinion”. Here, rewards are given when individuals question the majority assumption and accurately predict an innovation few others saw. Wrong predictions and common predictions receive the same reward: nothing46. Minority opinion forces individuals to explore concept spaces far away from others, creating greater information value for the community. This incentive structure increased collective intelligence by 20% compared with market incentives and 40-50% compared with simple rewards.
In Socos’s framework, minority opinion incentives work together with incubation and titration to drive exploration within and across cells and maximize the community’s collective intelligence. Matchmakers inherit the same rewards as the cells they manage, encouraging them to diversify “bets” across a concept space. However, this entire scheme has the potential to produce unintended consequences. For example, scientific publishing has a well-known winner-take-all problem in which groups that are the first to publish (or often just the first to be recognized) receive all of the credit despite building on the insights of an entire field. This often induces scientists to be secretive about their research and inhibits sharing of data and methodology across cells. While minority opinion is not winner-take-all, only a minority will receive rewards for any given innovation. This presents a challenging problem of how to motivate the entire community when, by definition, the majority receives nothing and their unadopted discoveries are discarded. Instead, rewards should be given out, not only to the cell and cluster producing an innovation, but also distributed to the entire foundation of insight that led up to the discovery. With asynchronous tools and concept mapping, we can understand how the inventing cell relied on past research and spread the reward back through the entire chain.
Think of it as “git-for-science”, where cells openly share hypotheses, methodologies, and discoveries to be forked and remixed by other cells. If a given repository ever produces an adopted innovation, credit and rewards are shared back through all of the contributors. In this way, unadopted discoveries contribute to the community’s collective intelligence and their originating cells are still incentivized.
Putting our framework all together, innovation engineering cycles through phases of incubation, maturation, and dissemination. Incubation launches the cycle with small, flat cells of diverse collaborators exploring concept spaces for new ideas. The maturation phase brings cells together into clusters to develop promising ideas into full practices. Finally, during dissemination, clusters coordinate communications to spread innovative practices throughout a community. Across all of these cycles, the matchmaker leverages tools like personality models and dynamic concept maps to remix collaborations and titrate interactions. They play the crucial role of maintaining the allostatic balance of trust and novelty within the microstructure of cells and across the macrostructure of the entire community. This complementary diversity combines with minority opinion incentives to maximize collective intelligence. Where the random chance of density=serendipity drowns out much of the very innovation it’s meant to facilitate, innovation engineering is an intentional practice that lifts the existing capacity of any organization.
You should stop relying on dumb luck and watercooler conversations as your source of innovation. Adopt our framework today or explore one of your own given everything you’ve just learned. As you do, you will discover one last major source of friction to innovation previously masked by the noise of density=serendipity. Throughout our framework, you can see the key role of complementary expertise, minority opinions, diverse concepts, and novel collaborations. The greatest innovation requires the greatest diversity at all scales. This brings us to the Diversity-Innovation Paradox.
Diversity-Innovation Paradox
I’ve already noted the large experimental research literature on the role of diversity in creative problem solving and collective intelligence. In fact, within science itself, simply adding new collaborators increases originality, innovation, and multidisciplinary impact, in part by restoring the allostatic tension between trust and novelty. Research like this extends these findings well outside of lab-controlled experimental conditions, and this phenomenon extends beyond scientific innovation. Many years ago, I was on an advisory board for Credit Suisse, the Swiss banking giant, when we released a report showing that corporate boards with at least 1 woman had 3% greater return to shareholders, and that boards with 3 or more women returned an average of 5% more47. Research tracing the cultural diversity of thousands of London companies found that diverse management teams, in terms of both ethnicity and immigration status, produced more product innovations than their competitors. Even in the unsexy world of fishery ecosystems, diverse groups better understood “complex feedbacks and interdependencies” than homogenous groups. Just as with our matchmaker framework, however, the diverse inputs of fishery stakeholders needed a proper aggregation process–simply throwing every idea and stakeholder together in a room produced no advantage.
From global corporate boardrooms to London entrepreneurs, from fishery ecosystems to scientific innovation, increased diversity brings increased value creation. Still skeptical? What if we looked at all 1.2 million doctoral dissertations published in the last 50 years? This is exactly what was done in one of my favorite papers of 2020. Using text analysis and machine learning, the authors analyzed the career trajectories of every US doctoral recipient since 197748. They found that “demographically underrepresented students innovate at higher rates”. Unfortunately, this wasn’t the paper’s only finding. Those novel contributions from underrepresented scientists were less cited than their peers’. Despite contributing greater innovation, underrepresented scientists were less recognized and had worse career outcomes, robbing us all of their future impact. This phenomenon is known as the diversity-innovation paradox and it represents a major drag on global innovation.
Untrusted
While this paradox has deep roots in history, institutions, and personalities, it is fundamentally about trust49. As I’ve repeatedly argued, trust and diversity play fundamental and opposing roles in innovation and distributed cognition. Our well documented preference for trust over diversity drives organizations into the paradox. For example, cognitively diverse teams are more effective, and yet coworkers tend to sort themselves into cognitively homogeneous groups over time50. Even when an individual has a clear incentive to share information with a collaborator, they share less with individuals that are more culturally dissimilar. Left unchecked, these biases foment cultural barriers that suppress cognitive and broader diversity.
As cultural norms begin to favor homogeneity, individuals begin to conform to the norms of their ingroup “even when they understand that the norms…are arbitrary.” Deeper still, their emotional responses shift to align with their perceived ingroup. Although there is significant variation in how individual brains process social signals around trust51, a pattern emerges in which ingroup trust is associated with reward circuits while outgroup trust requires effortful top-down control. Additional stressors, such as time or financial pressures, increase the top-down effort needed for outgroup trust while having no impact on the reward circuits for ingroup trust. This creates another bias driving us towards homophily and undermining the prosocial behaviors necessary for success in diverse teams (and societies).
These barriers can be overcome, however, with engagement. Increased interpersonal experience shifts interpersonal trust from effortful medial prefrontal control to more reflexive (i.e., “automatized”) brain circuits. Simple engagement with people of different races and genders reduces belief in stereotypes and other outgroup associations. It is only when trust and diversity are brought into balance that teams can avoid the Paradox and gain the full innovation potential of their community.
Without that balance, lack of trust dominates and robs us of capacity. For example, in many academic fields, including economics and the sciences, seminar audiences are systematically less trusting of women, asking more total questions and exhibiting more patronizing or hostile attitudes, even after controlling for differences in quality of work or field of research. In the world of entrepreneurship, investors of all genders strongly prefer pitches from attractive male entrepreneurs over female entrepreneurs, even when the pitches are otherwise identical.
Analysis of meetings between venture capitalists (VCs) and female startup founders reveal that VCs undermine female founders with questions about losses while supporting male founders with questions about gains, and later negatively describe qualities in female founders that receive praise in male founders (e.g., “lacks experience” vs. “full of potential”). This difference in trust persists despite the finding that female founders return more than twice as much per dollar invested as male founders.
The potential anonymity of remote work suggests one possible solution to these known biases: we could all hide our differences within digital personas. Behind avatars and chat handles we could all discard our identities and hide our differences. This has appeal for many, but rather than removing difference, all of the research above suggests that it would actually produce the opposite, exaggerating even subtle differences and worsening ingroup-outgroup effects. Trust grows with engagement, not anonymity.
As noted way back in “Measuring Remote Success”, employees with more facetime with managers receive more recognition and more promotions. For most, though, working remotely reduces social network centrality, meaning that the average remote worker is less present in the minds of their coworkers52. This increases the need to manage one’s impression amongst coworkers and leaders within the company. While everyone experiences this same increased need for “job-focused impression management”, managers treat this behavior more negatively in employees of a different gender. Without the reflexive trust given to those similar to you, career management looks conniving and deceitful. This even plays out in elite levels of American politics–male senators are seen more positively the more they talk, while female senators…never know when to stop persisting.
“Distributed Innovation” explored how majority influence and herding effects reduce collective intelligence and innovation, and revealed the powerful impact of minority opinion incentives. This same effect is further exaggerated in situations demanding ingroup-outgroup trust. For example, when a deliberative process uses majority rule and few women are present, women experience more interruptions when speaking and their contributions are less trusted, reminiscent of the female academics described above53. When groups are required to respect minority opinion (e.g., unanimous rule), women are actually interrupted less than men, as the incentives of the system demand an effortful allocation of outgroup trust54.
In “Controlling the Beyond-Control” I described how the attribution bias causes us to arbitrarily associate situational challenges to perceived failings of specific individuals. In the context of trust, it is clear that negative attributions become even more pernicious across group divides. Even location plays a role in exaggerating attribution bias as more distant collaborators receive more blame for failures, often hiding the real source of a failure and allowing it to persist. And for all our generic complaints of “Zoom fatigue”, challenges with gaze and space in video meetings disproportionately affect those in outgroups. Cultural differences affect how we contextualize and interpret eye contact and other displays of emotion, influencing the way people interact. For example, East Asians tend to perceive direct gaze as evidence of anger or sadness compared to Western Europeans. Even visual cues like the perceived distance or relative position of a face in an image affect perceptions of gender and emotion. And those perceptions in turn alter the neural circuitry of trust.
Even within America, factors such as differences in family size or self-expression can easily fuel attribution bias. For example, census data shows that Black families have 20% more people in a household than the average US family; Mexican-American families are 80% larger. This creates more competition of bandwidth and less private space for video meetings. That little extra background noise or choppiness in video quality can easily lead to false assumptions about competence. All of this while that same video feed intrudes into a private home of those in outgroups, exposing “formerly safe, private spaces” to scrutiny and ingroup judgement.
Simple access to remote work is a form of privilege many don’t fully appreciate. Even before the pandemic began, there were racial biases by income and industry in who was able to work remotely. Remote work also exacerbates broader socioeconomic and regional differences. Despite significant variation, remote “jobs typically pay more than jobs that cannot be done at home and account for 46% of all US wages.” Of the highest earners in the US, 71% report being able to work from home, compared to only 41% from the bottom quintile. All of these differences in access further reduce the connections across socioeconomic, racial, and regional divides. In the end, you are more likely to encounter certain kinds of people working remotely, reinforcing in our minds that elite work is largely white-ish, male-ish, highly educated, and culturally homogeneous. The very tools we use to stay connected tend to exaggerate ingroup-outgroup effects and bolster the Diversity-Innovation Paradox.
Even the received wisdom of those with remote work experience reveals deep assumptions about the nature of trust and collaboration. “Set the meeting to 15 minutes by default, and only make it longer if absolutely necessary (the shorter the meeting, the more succinct you will have to be, and the less time there will be for pointless small talk and rambling).” A culture of 15-minute scripted meetings is a culture of homogeneity. Where is the trust in the assumption that others will waste your time? This idea might hold for rote cognitive labor where there’s no need for chit chat, but in creative labor this mindset reinforces ingroup-outgroup divides and reduces innovation.